AI Is Redefining the Role of the Scientist – Here’s How
AI and lab automation are shifting scientists' roles from manual work to more analytical, strategic and creative tasks.
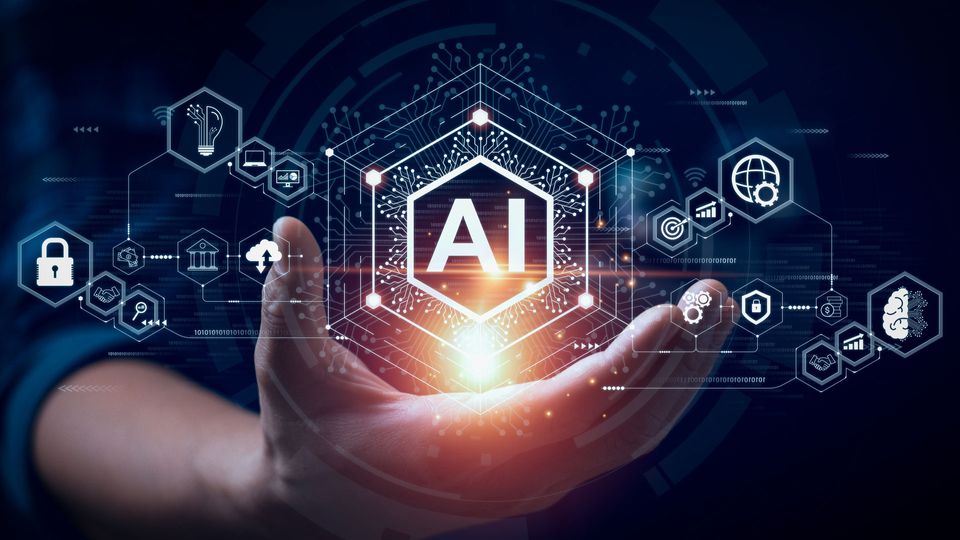
Complete the form below to unlock access to ALL audio articles.
The integration of artificial intelligence (AI) and automation is transforming laboratory research by enabling entire workflows to be automated end-to-end, with modern labs equipped with various instruments capable of performing predefined tasks. In cell line development, for example, there are tools to automate liquid handling, incubation and imaging. With AI, these processes form a seamless pipeline, reducing the need for manual oversight. This all-in-one process dramatically improves overall efficiency and scalability, making assays more reliable and reproducible.
AI also helps scientists acquire high-quality data in the shortest possible time and avoid making mistakes that would result in time wasted redoing the experiment. Through chat interfaces, users can clarify instructions, and AI can suggest settings based on past samples. Further, AI can help by presenting the data in an understandable format, making it more interpretable so scientists can decide what to do next.
However, scientists must be cautious. Over-reliance on AI may weaken critical thinking and troubleshooting skills. Biases in AI models can introduce errors, making human oversight crucial to maintaining research integrity, transparency and reliability.
How AI integration is changing the traditional role of scientists
By automating simple decision-making processes, valuable time is gained to focus on more meaningful and complex tasks. This allows scientists to become more creative with their research and work more efficiently. Through handling routine and tedious tasks, AI enables researchers to concentrate on critical aspects of their work, such as analyzing data, interpreting results and making informed decisions.
Moreover, with the time saved, scientists can dig deeper into innovative research areas, explore new hypotheses and design more sophisticated experiments. This newfound freedom fosters a more dynamic and creative research environment, leading to groundbreaking discoveries and advancements in their field.
While AI and automation do offer some freedom to focus on other tasks, it's not a completely hands-off, self-driving approach, and scientists still need to monitor the process as relying solely on AI could lead to false assumptions and incorrect decisions. They must make decisions in scenarios where AI has not been trained, for example. At this point, scientists must stay involved and informed about events that may require their input.
Do scientists need a new skill set?
To ensure maximum efficiency of automation and AI integration into a laboratory, researchers should be proficient in using AI-enabled, high-content screening systems for robust image and data analysis and, ultimately, for automating decision-making processes. This involves understanding where AI can provide immediate assistance – such as in data processing and preliminary analysis – and where it still requires guidance from research experts, particularly in interpreting complex biological phenomena.
By recognizing the strengths and limitations of AI, researchers can effectively integrate these tools into their workflows, ensuring that AI complements their expertise rather than replaces it. Together, these skills enhance the overall efficiency and effectiveness of laboratory work, enabling researchers to handle complex biological problems with greater accuracy and confidence.
Further, AI-driven segmentation is crucial for accurately identifying biological objects throughout experiments. Researchers must be adept at training AI models to recognize and accurately segment these objects robustly across various phenotypes, including different growth stages, cell cycle states and biological contexts. This foundational skill is essential for ensuring precise identification and interpretable results. Honing skills in generating and curating training datasets – as well as using AI tools to automate and enhance this process – will be crucial for ensuring the high quality of AI-generated results.
Ethical considerations and associated challenges
AI is only as good as the data it has been trained on. Ensuring that AI systems are trained on comprehensive and diverse datasets is critical for their accuracy and reliability. Additionally, continuous monitoring and updating of these datasets are necessary to adapt to new information and evolving research needs. If the input training data does not include all the relevant data for a particular experiment, you might end up with incorrect results.
Over-reliance on AI can lead to false conclusions, which is where ethical considerations come into play. This is why the importance of responsible AI is emphasized. Responsible AI involves transparency in how AI systems make decisions, allowing researchers to understand and trust the outcomes. By maintaining a balance between AI, automation and human oversight, we can leverage the strengths of AI while mitigating its limitations. This approach enhances the quality of research and upholds ethical standards, ensuring that AI serves as a valuable tool in scientific discovery.
Despite advancements, AI still has limitations. For instance, AI can handle simple tasks, but it may struggle with more complex assays and samples due to insufficient information on how to treat them. This is where scientists can step in and apply their expertise to make necessary adjustments, ensuring that the results are accurate and relevant to a specific assay. As long as scientists understand AI’s capabilities and limitations, they can effectively harness it to catalyze their next discovery.
Shaping the future of laboratories and research scientists
The future of AI/automation integration and the role of research scientists will be a collaboration. Although scientists' future roles may change, they will still be centered around teamwork in the coming years. AI will continue to interpret certain data or assist with experiments, while scientists decide what to do next. This collaboration will allow scientists to leverage AI's capabilities to handle large datasets and complex analyses, freeing them to focus on innovative research and strategic planning.
Once AI is trained on a wealth of consistent data – a current limiting factor – productivity will increase even further. Ensuring the integrity and reliability of the data used for training AI is crucial for its effectiveness, otherwise, it could lead to false conclusions for scientists. As AI systems become more sophisticated, the importance of maintaining rigorous standards for data quality will only grow, ultimately enhancing the accuracy and utility of AI in scientific research.