AI, MPS and the End of Animal Testing?
Dr. Thomas Hartung discusses how AI and organs-on-chips are transforming drug testing.
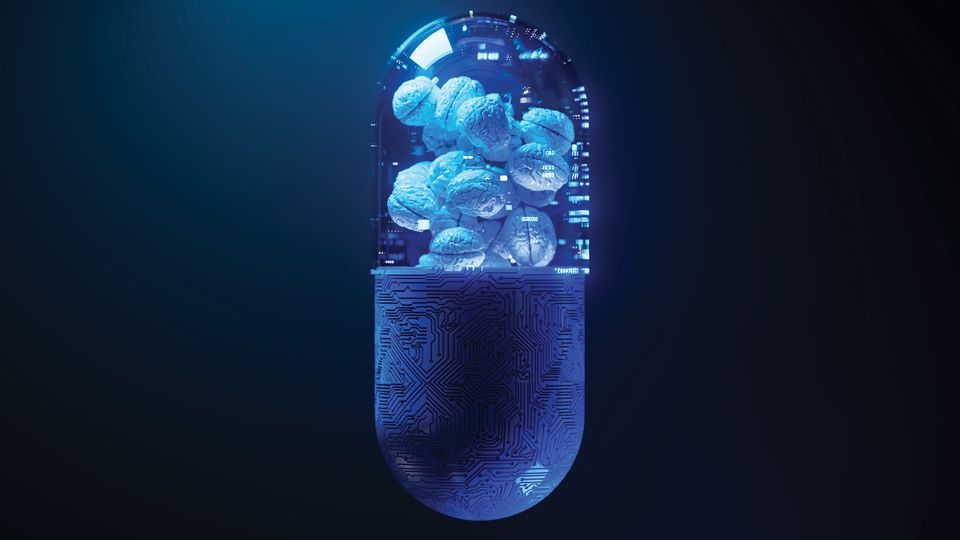
Complete the form below to unlock access to ALL audio articles.
For decades, traditional animal testing has been the gold standard for evaluating drug safety, but a growing body of evidence suggests that these methods may not always provide the most accurate predictions for human responses.
New technologies such as artificial intelligence (AI) and microphysiological systems (MPS), i.e., human cell-based models, are emerging as powerful, evidence-based alternatives.
Dr. Thomas Hartung, a professor and the Doerenkamp-Zbinden Chair for evidence-based toxicology at Johns Hopkins Bloomberg School of Public Health, leads research in non-animal approaches to drug testing, influencing both scientific progress and regulatory policies. At WORD+ 25, he delivered a talk titled "Evidence-Based Animal Alternatives for Drug Discovery", where he explored the urgent need for more human-relevant models in pharmaceutical development.
Hartung described AI as "a tremendous revolution that is taking place in the way we are dealing with information”. He highlighted the explosive growth of global data, which now doubles every 18 months, far outpacing the growth of computing power, which has doubled every 24 months over the last 60 years. Hartung also pointed out that, since the rise of deep learning in 2010, AI has been doubling in capacity every three months – a shift that is redefining the speed and scale at which scientific discoveries are made.
Technology Networks caught up with Hartung after the event to discuss his insights on the future of drug testing, the role of AI in toxicology and the regulatory and ethical challenges in moving away from animal models.
What are “evidence-based alternatives” to animal testing in drug discovery, and why is this approach gaining traction?
Evidence-based alternatives to animal testing represent a modern, systematic approach to testing drug safety that relies on human biology-based methods combined with rigorous scientific evidence. Just as doctors use evidence-based medicine to make the best treatment decisions for patients by carefully reviewing all available research, we can apply similar principles to develop and validate new drug testing methods that don't rely on animals.
These alternatives include:
- Human cell-based models grown in labs
- 'Organs-on-chips' that mimic how human organs function
- Advanced computer modeling
- Analysis of molecular pathways involved in toxic reactions
The traditional animal testing methods developed in the 1950s have never been systematically evaluated for how well they actually predict human responses. When we carefully examine the evidence, we often find significant differences between how animals and humans react to drugs.
New technologies now allow us to study drug effects directly in human cells and tissues, providing more relevant information about potential safety issues in humans. The FDA's 2022 Modernization Act specifically recognizes the value of these human biology-based approaches.
Evidence-based alternatives help address the massive challenge of evaluating the growing number of new chemicals and drugs. By using systematic, transparent methods to assess safety, we can potentially get safer drugs to patients more quickly and efficiently.
This transition represents a revolution in how we conduct safety testing – moving from reliance on animal models to an evidence-based approach that prioritizes human relevance and scientific rigor.
MPS, such as organs-on-chips, represent a significant advancement in drug development by recreating key aspects of human biology in ways that traditional cell cultures and animal models cannot. These systems combine sophisticated engineering with cell biology to create three-dimensional tissue architectures that can incorporate multiple cell types, tissue interfaces, fluid flow and mechanical forces that mimic human physiology.
MPS can better predict how drugs will affect humans before clinical trials begin, helping to identify potential safety issues or lack of efficacy that might not be caught by animal testing. For example, they have successfully detected dangerous blood vessel toxicity that was missed in animal studies but later caused clinical trial failures. MPS are particularly valuable for testing highly engineered therapeutic molecules that don't interact with animal targets, which represent more than 50% of the new drugs coming to the market, making traditional animal testing impossible.
Additionally, MPS can be personalized using cells from individual patients, enabling precision medicine approaches that could predict how specific patients might respond to treatments. This is especially valuable for rare diseases where large clinical trials are difficult to conduct.
However, we are not yet at the point of completely replacing animal testing with MPS. While some MPS have reached the level of reliability needed for internal decision-making in pharmaceutical companies, none have been formally validated for regulatory drug approval processes. The field still needs to demonstrate that MPS can consistently produce results that meet regulatory requirements. There are also ongoing challenges in scaling up production and ensuring the robustness of these systems.
AI excels at data integration and analysis. These systems can analyze vast amounts of heterogeneous data from multiple sources including animal studies, epidemiology, clinical reports, in vitro assays and various omics approaches. This allows scientists to retrieve and extract more value from existing data rather than requiring new animal tests. Machine learning techniques can identify patterns and relationships across diverse datasets that might not be apparent through traditional analysis.
AI also offers powerful predictive capabilities. Deep learning models can be trained on existing toxicity data to predict hazards for new chemicals without requiring additional animal testing. These AI models can learn from both chemical structural features and bioactivity data to make more robust toxicity predictions. Modern AI systems have shown performance comparable to or better than traditional animal tests in some areas, like predicting liver toxicity and developmental toxicity.
AI helps analyze complex biological pathways and mechanisms of toxicity, enabling better understanding of how chemicals affect human biology directly, rather than relying on animal models that may not perfectly translate to humans. It can integrate pathway-based approaches that are referenced to human biology, minimizing the need for species extrapolation.
It also brings significant improvements in efficiency and speed, rapidly screening large numbers of chemicals for potential toxicity, which is much faster and more cost-effective than traditional animal testing approaches. Automated systems guided by AI can process and analyze data in real-time.
AI also offers important ethical benefits by reducing reliance on animal studies. These tools help address ethical concerns around animal testing and support the 3Rs principle – replacement, reduction and refinement – in toxicology. AI methods can often provide more comprehensive safety data while using fewer or no animals.
A major regulatory challenge is that current drug approval processes still expect and require animal testing data before allowing human trials to proceed. This creates a catch-22 situation where companies must conduct animal studies to move forward, even when alternative methods might provide better human-relevant data. To overcome this, regulatory agencies need to demonstrate more flexibility in accepting validated alternative methods and create clear pathways for their approval.
The pharmaceutical industry faces unique pressures that affect this transition. With drug development already costing around $2.4 billion and taking 12 years on average, companies are hesitant to deviate from established testing paradigms that regulators accept, even if they recognize the limitations of animal models.
Addressing this requires building sufficient evidence that alternative methods can reliably predict human responses while maintaining or improving development efficiency.
New approaches like MPS and AI-based models must prove they can consistently reproduce or improve upon animal study results. This requires extensive validation studies comparing alternative and traditional methods, which takes considerable time and resources. Overcoming this challenge needs coordinated effort between industry, academia and regulators to generate validation data.
From an ethical perspective, while reducing animal testing is a key goal, there's also an ethical imperative to ensure new drugs are adequately safety-tested before giving them to humans. Finding the right balance between reducing animal use and maintaining rigorous safety standards is crucial.
The future of drug discovery is likely to involve a gradual but significant transformation rather than a complete paradigm shift within the next decade.
The field is moving toward an integrated approach that combines several key technologies. MPS or organs-on-chips will play an increasingly important role by recreating aspects of human biology more accurately than traditional cell cultures or animal models.
AI is becoming a transformative force in drug development. While it won't completely replace other methods, AI is becoming an invaluable 'copilot' in the drug discovery process, helping to identify patterns and predict toxicity more efficiently than traditional approaches.
However, a complete shift away from animal testing within the next decade appears unlikely.
Regulatory requirements still mandate animal testing data for drug approval in most cases and while there's movement toward accepting alternative methods, changing these deeply embedded regulatory frameworks takes time.
Some complex biological interactions still can't be fully replicated by current alternative methods. Living systems are complex and we'll likely need animals for certain aspects of testing until technology advances further.
Even with perfect alternative methods, there would be a significant transition period as companies adapt their processes and regulators gain confidence in new approaches. The pharmaceutical industry, given its high stakes and costs, tends to be conservative in adopting new methods.
The key to accelerating this transition will be generating sufficient evidence that alternative methods can reliably predict human responses, while developing clear regulatory frameworks for their acceptance.
I had the privilege of developing and pushing several alternative methods with my team. In my PhD, I developed a coculture of liver cells with immune cells to study inflammatory processes, which is now quite widely used in the pharmaceutical industry.
I then developed a pyrogen test, which is used to find microbial contaminants in drugs for injection and infusion. I then led the validation study for mine and some similar tests. These tests are now increasingly accepted and replace a rabbit test. In Europe alone, this saves 150,000 rabbits every year.
At Hopkins, we are best known for our brain organoids – we were the first to mass-produce such MPS with many research applications. Our AI models are groundbreaking – Australia was the first to accept them in their Chemical Legislation.
However, research can only serve as proof-of-principle. I think the most important work is in organizing the field in conferences, guidance documents, education, consensus building and advising policymakers.