AI’s Next Horizon: Cell and Gene Therapy Manufacturing
To improve CGT manufacturing scale-up, AI will need better data.
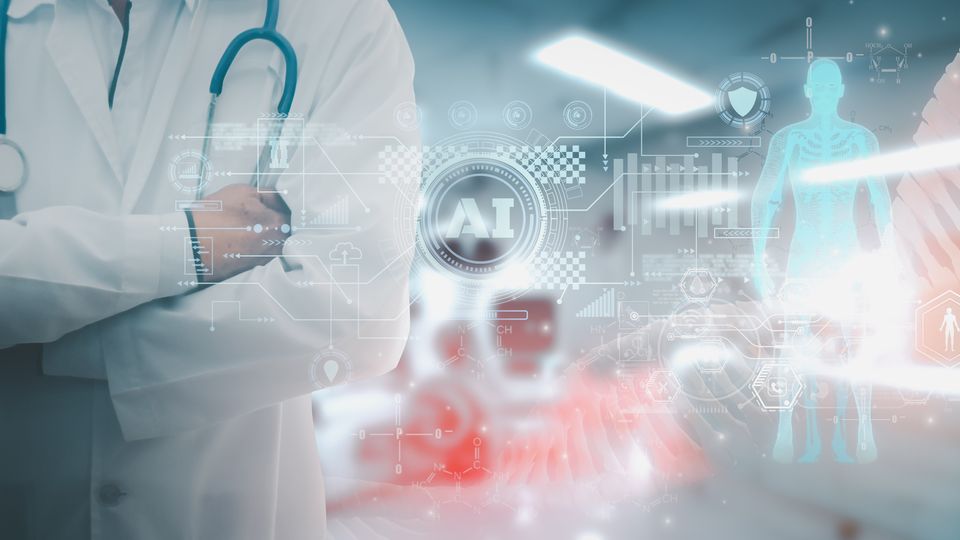
Complete the form below to unlock access to ALL audio articles.
Despite the perception of biopharma as a field that is slow to adopt new technology, artificial intelligence (AI) has been quietly improving the sector for years, from candidate prioritization in drug discovery to adverse event triage in clinical trials. Still, there are plenty of remaining pain points where improvements are desperately needed. One of these is cell and gene therapy (CGT) manufacturing.
Cell-based therapies are made from material drawn from donors or from patients themselves, with often complex logistics needed to move starting material to the site of manufacturing and engineered cells back to a patient, and a protracted cell modification and expansion process in the middle. This results in processes that today are too expensive to be scaled efficiently, and so slow that patients face dire consequences while waiting for treatment.
For each step along the way, AI has the potential to accelerate processes and reduce costs. The challenge is that more data is needed to train the algorithms that are needed to optimize and ultimately help standardize the relatively young CGT space. Existing data is often inaccessible, due for example to outdated collection and storage methods. Beyond this, new data will need to be generated and stored. Doing so will rely on new technology like cutting-edge biosensors and sufficient infrastructure to make it all available where AI can be leveraged.
Changing from the manual
The speed of the personalized CGT revolutions has been remarkable. But as a result, we are still experiencing growing pains and bearing the marks of early development. Today CGTs are largely personalized, autologous therapies, where traditional large-batch manufacturing doesn’t factor in. Production processes often come directly from work connected to academic discoveries, which results in a limited initial focus on scalability.
As a result, processes are frequently cumbersome, heavily manual and typically paper-based – as is data capture. Any use of AI – or even less sophisticated methods of standardization and optimization – therefore requires digitization as a first step. However, this is rarely a straightforward matter, and developing new processes comes with costs that early-stage therapy developers may not have the resources to support.
This is further complicated by the newness of the field. We are still learning which are the most relevant parameters to collect in order to improve outcomes. That means we also still must sort out the right infrastructure to support this data collection and analysis.
But efforts are beginning to pay off. The industry has recognized the need for standardization, and digitization is now often embraced early as a necessary step of CGT process development, paired with at least plans for automation. Additionally, the need for more and better data across the board has inspired a series of technological advances, partnerships and maturing conversations that are helping us move towards optimized and standardized processes – and ultimately, quicker, less expensive manufacturing
More data from more sources
Ongoing work demonstrates the potential for integrating AI into CGT manufacturing, when the right data is available.
At the ISCT 2024 conference, researchers shared results from a study using a machine learning (ML) model to analyze and optimize cell culture parameters. In the study, the team leveraged an ML algorithm to generate high T-cell densities in a miniature bioreactor. They also uncovered a metabolic model that could reduce the cost of goods by as much as half through reagent efficiency.
For true optimization, much more data must be collected to train algorithms to identify the most important parameters and predict the impact of changes during cell culturing. The key to unlocking this level of optimization for CGT manufacturing is increased collection of biosensor data with advanced tools.
Continuous biosensor-based monitoring can help characterize critical metabolic cell culture parameters such as lactate and glucose. This includes on-line sensing, where parameters are monitored in parallel with manufacturing, and at-line sensing, where real-time monitoring is possible as samples are taken directly from the manufacturing processes. Based on these parameters, computational prediction algorithms can then be deployed to determine the number of cells that will be produced, as well as the timing of cell harvest.
This is the Industry 4.0 approach: by combining advanced monitoring with automated platforms, the computational models can enable better real-time decision-making across multiple processes simultaneously. Taking it one step further, this can also improve efficiencies by enabling off-site monitoring of the production of multiple cell therapies at once, from a distributed network of sites.
Partnership is another important piece of the puzzle. The makers of automated cell therapy manufacturing platforms have begun working with biosensor companies to integrate the tools necessary to measure both the inputs and outputs of multiple steps.
The combination of technologies gives CGT developers the ability to do automated sampling and integrate the vastly expanded data flow to better understand how to impact outcomes, with potential new prediction models guiding them toward new operational efficiencies. Optimizing individual processes will be tremendously valuable but could be outshined by opportunities to develop industry-wide standards based on the pooling of data.
This will require broadening the types of data we can collect to include every part of the CGT production process, and leveraging partnerships with developers, academics, apheresis centers and providers.
As one example, we have already seen this potential while working with researchers at Boston Children’s Hospital and Harvard Medical School. Together, the team leveraged data from apheresis platforms used to collect material from the blood of patients with sickle cell disease (SCD).
Apheresis can be used during various parts of a patient’s SCD journey, including as part of regular red blood cell exchanges. Demand for apheresis for this population has grown recently in no small part due to the recent approval of two gene therapies, and it has played an important role in the development of these therapies – and doubtlessly, will be important for others in the future.
Patient cells that are to be gene-modified or edited are collected using apheresis. However, the viscosity of blood from people with SCD can lead to unique difficulties during apheresis, including clumping. The academic researchers used data from our devices to significantly improve cell yields, with potential implications for the manufacturing processes. Through training and educational programs, we have begun sharing these learnings more broadly.
Given that data flow is expected to increase everywhere processes are automated – from logistics to fill and finish – there will be opportunities to use AI to help us establish standards that can raise quality and reduce costs throughout the field.
Data infrastructure
In addition to quantity, the quality of data helps determine the ceiling of AI’s capabilities. Because of CGT’s manual, academic lab history, data infrastructure has only recently become a priority. But developers are now building the kind of data environments intended to ensure proper capture of the new and increasing data flows.
The industry has come to recognize there are both unintended and intended obstacles for the kind of data sharing that will enable AI to help everyone move toward standardization. Too much data is difficult or impossible to use; in some cases, this results from paper records that have yet to be digitized. In others, this is related to how data is housed.
For example, data may be housed in systems with compatibility issues, meaning that even data sharing within an organization is not possible. Ensuring these datasets can interact, and be made available between companies as well, also requires consistent structuring that can be easily parsed by an algorithm.
Ongoing and new data capture must similarly conform to a standardized format. In addition, these companies often partner with many developers and can facilitate data share, meaning enabling technology companies must be part of the conversation.
In parallel, certain attitudes toward data sharing must change for the field to progress quickly. CGT process development is particularly guarded, even for biopharma, given that many of these therapies are based on bespoke processes. Data sharing always requires consideration of IP concerns – but particularly in this space, where it is unclear which parameters have the biggest effect on outcomes, it can be difficult to know what is appropriate to share.
AI is still so new in this space that few companies have the internal expertise to prioritize the kinds of changes to data infrastructure and sharing that will be needed. Also, the regulatory landscape connected to AI is still evolving and will rightfully prioritize the ethical protection of patient privacy.
Still, it’s easy to see why an industry with such broadly shared challenges has more to gain through partnership and data democratization than siloing. Companies with commercial therapies are struggling to make them fast enough or affordably enough to reach all the potential patients in need. The next generation of therapies have common challenges as well, whether that is adapting automated platforms, scaling up a new cell type, or moving toward distributed manufacturing models.
It is important as a field that we remain committed to solving the data access problems, and pursue this through increased partnership and integration, between and across organizations.