Organoids, Beyond the Hype
How UC San Diego’s HUMANOID™ Center is engineering solutions to prevent an unparalleled era of reproducibility crisis in biomedical research

Complete the form below to unlock access to ALL audio articles.
Reproducibility is a buzzword in the world of scientific research. Frequently, it is followed by the jarring, but often apt, term “crisis,” to describe the dangerous implications that emerge when one scientist is unable to reproduce another’s work. Perhaps nowhere is reproducibility more integral to a study’s relevance and success than in biomedical and translational research. After all, these are areas of research that seek to model humans themselves – their individual organs, their intricate metabolic processes and the diseases that befall them. If the outcome of a study involving human biology is not reproducible, then neither is it relevant on any population-based level.
Many scientists agree, unfortunately, that it is precisely these areas of research in which reproducibility and translational relevance are seriously lacking. For decades, animal models have been the linchpin both of basic biologic research and of preclinical drug testing and development. However, it is increasingly evident that rodents – and even primates – are not human, and their capacity to unravel the mechanisms of diseases like Alzheimer's, inflammatory bowel disease and various cancers, or to reliably predict the effects of novel compounds in humans, is inherently limited. Despite unprecedented advancements in computational power, speed and precision (evidenced most recently by the remarkably rapid development of the COVID-19 vaccines), success in drug discovery lags far behind expectations. Approximately 90% of drug candidates fail clinical trials – all of which passed toxicity and efficacy testing in animals. More specifically, 40–50% of candidates fail efficacy benchmarks related to disease heterogeneity and patient diversity, and about 30% fail due to unmanageable toxicities.1 In 2013, Dr. Elias Zerhouni, a former National Institutes of Health (NIH) director, remarked: “We have moved away from studying human disease in humans. We all drank the Kool-Aid on that one, me included...The problem is that [animal testing] hasn’t worked, and it’s time we stopped dancing around the problem...We need to refocus and adapt new methodologies for use in humans to understand disease biology in humans.”2 This honest admission reveals just how vital new methods of disease modeling are to the success of modern biomedical research.
The birth of organoids
Enter Dr. Hans Clevers, the father of organoids. In 2009, Clevers and his colleagues pioneered a revolutionary method of creating miniature three-dimensional (3D) models of human organs grown from stem cells – what he termed organoids. Organoids can be grown either from induced pluripotent stem cells (iPSCs) or patient-derived stem cells. iPSCs are immortalized cells derived from a single source that can be reprogrammed to mimic nearly any organ, while patient-derived stem cells are cells isolated directly from patient tissue that maintain the identity of the organ from which they were collected. Either way, organoid models harness the incredible regenerative power of stem cells to proliferate, organize and function in ways that can accurately recapitulate the behavior of their endogenous environment. As a result of this unique potential, they are already permanently changing the drug discovery sphere. For diseases where clinical trial recruitment is particularly challenging, such as those caused by rare genetic mutations, organoids have proven invaluable. One such disease is cystic fibrosis, sometimes caused by a rare homozygous mutation in protein folding and ion channel function. Patients with this genetic background have experienced incredible results from a combination treatment first tested on patient-derived organoids (PDOs) with their exact mutation.3 This groundbreaking speed in drug discovery caught global attention, including coverage in leading journals such as Science Magazine.4 Since then, many similar success stories have emerged, with organoids paving the way for drug development in rare diseases.
The impacts of organoid models are not limited to personalized medicine, and in fact extend beyond these specific models into broader endeavors such as drug repurposing. Recent studies have utilized collections of organoids from different patients of a specific disease type to screen for sensitivity to novel compounds, drugs in clinical trials or those that have been approved for use with a different indication. This has enabled the discovery of compounds with specific antitumorigenic properties relevant to cancers largely insensitive to current standards of care. Esophageal cancer, for example, can often have devastatingly little response to traditional chemotherapy. Indeed, a recent screening of esophageal PDOs revealed very limited sensitivity to approved chemotherapy combinations, but some promising response to an inhibitor traditionally used in the treatment of melanoma.5 Without the valuable insight of organoid models, the prescription of this compound would likely have been limited to the type of cancer for which it was originally approved.
Perhaps the area in which the superiority of organoid models over traditional animal studies is most evident is in preclinical toxicology research. Although most drug candidates fail Phase 3 clinical trials due to failure to meet efficacy endpoints, a significant percentage in all phases also fail due to unforeseen toxicities. Recent reports suggest that using animal models to predict toxicity in humans is akin to the flip of a (very expensive) coin. An analysis of over 2,000 drugs that entered clinical trials shows that, while demonstrated toxicity in animals such as mice, rats and rabbits can be correlated with associated toxicities in humans, the absence of a toxic effect in these species has virtually no correlation to the lack of an adverse response in a human study participant.6 Hepatic organoid models, on the other hand, have been shown to predict cell viability and cholestatic or mitochondrial toxicity for over 200 approved drugs with sensitivity and specificity levels near 90%.7 Maintaining high predictive values is vital, for unforeseen toxicities in clinical trials are not only devastating to the success of the trial, but also dangerous for the involved participants. Organoids have the potential to mitigate this risk significantly. Recently, a liver-on-chip system lined with hepatic organoids correctly predicted the toxicity of Tenofovir-Inarigivir, a combination antiretroviral treatment indicated for both hepatitis B virus (HBV) and human immunodeficiency virus (HIV) that eluded hepatotoxicity discovery until Phase 2 trials.8 If this model had been used preclinically, the dangerous toxicities suffered by human participants could have been avoided.
Unprecedented potential is not without unprecedented challenge
The potential of organoids to improve the reproducibility of biomedical research, the success rates of clinical trials and the translational relevance of lab-based discoveries is beginning to be recognized not only by the scientific community, but also by larger governing bodies. In September 2022, the United States Food and Drug Administration (U.S. FDA) passed the FDA Modernization Act 2.0, a landmark bill that removes the requirement for animal testing in the approval of any drug candidate that advances to clinical trials.9 The bill officially recognizes what the FDA has termed microphysiological systems (MPS) as viable alternatives to animal testing in preclinical research. MPS can include organ-on-chip studies, zebrafish models and in silico approaches as well as organoid experiments. This legislation, however, did not arrive with any formal guidance on the use of organoids as preclinical models. The Center for Drug Evaluation and Research (CDER) is just beginning to test 3D hepatic and cardiovascular models for drug toxicity determination. Emphasized by the organization is both the great potential of organoids and other MPS to be superior preclinical models and the critical need for quality control standards that ensure functional, robust and reproducible results.
Thus, there is little doubt that a significant milestone has been achieved in tackling unmanageable toxicity, a factor responsible for 30% of all drug failures. However, the challenge of the remaining 40–50% of failures, attributed to lack of efficacy, persists without robust guidelines, benchmarks or credible solutions. There is still no clear framework for leveraging organoids to test efficacy early in the drug development process, leaving this critical evaluation to Phase 3 trials and driving up the overall cost of drug discovery programs.
In fact, organoid technology contains a unique set of characteristics that challenge traditional methods of reproducible and quantitative data collection. Below are 10 specific effects that threaten the reproducibility of organoid research and decrease the ability of their models to predict drug efficacy consistently.
- Fingerprint effect: Organoids are as unique as the patients from which they are derived. Variability between healthy and diseased organoids, even from identical diagnoses, limits reproducibility. Addressing this requires large, diverse samples. Organoid studies are only as powerful as the size of the sample that experimenters are able to reach.
- Snowflake effect: Due to cellular heterogeneity, no two organoids, even from the same patient and grown under identical conditions, are exactly alike. This phenomenon makes reproducible and meaningful data more difficult to gather, especially when the focus is on high-content and not high-throughput experimental readouts (such as time-consuming and sophisticated imaging). Rigorous recordkeeping of items such as time in culture, passage number, media variety, growth conditions and freeze/thaw cycles can inform pooled samples of data before they are analyzed by statistical or computational methods, making reproducibility attainable. Additionally, quantitative imaging platforms designed specifically to improve throughput in organoid analyses are beginning to emerge.10
- Model drift effect: Over time, organoids can genetically and physically change in culture, losing their abilities to faithfully recapitulate disease state and their relevance as models. To prevent such deterioration in model fidelity, strict passage limits and regular monitoring for morphological (by automation in scaled up cultures) and transcriptional (by RNA sequencing) changes are essential. Use of biological media with the minimum concentrations of growth factors and additives necessary to sustain life can also ensure that organoids retain an identity as close as possible to the endogenous environment of their parent organ.
- Memory effect: Organoids derived from iPSCs lack the epigenetic "memory" of the tissues they aim to model. This distinction is critical, as epigenetic changes are now recognized as key drivers of most chronic diseases.11 For example, recent research demonstrated that iPSC-derived colonoids from patients with inflammatory bowel disease (IBD) fail to replicate the transcriptional profiles of colonic epithelial states observed in situ.12 Similarly, in cancer models, PDOs not only replicate the gene expression patterns of traditional two-dimensional (2D) cell lines, but also faithfully represent tissue structure, differentiation, intra-tumoral heterogeneity and microenvironment.13 Additionally, iPSC-derived lines such as liver models remain immature in enzyme profiles, rendering them suboptimal for toxicity prediction.
- House blend effect: The absence of formal FDA guidance or widely accepted standard operating procedures (SOPs) has led individual labs to develop unique methods for organoid culture. This variability in culture methods, protocols and media recipes undermines reproducibility, particularly when critical details remain unpublished. Inconsistencies in tissue acquisition (e.g., biopsy depth or type of forceps used), transport, isolation, culture conditions, freezing and recovery after thawing further complicate efforts to standardize models and to interpret results reliably. To address these challenges, it is essential to establish and adopt detailed, standardized protocols for every stage of organoid research. Publishing comprehensive methods in peer-reviewed journals should become the norm to ensure transparency and reproducibility. Standardization efforts must also extend to tissue acquisition, handling and culture procedures, with specific attention to minimizing variability.
- Territorialism effect: Restrictive consent forms and intellectual property claims often hinder collaborations and cross-validation studies, both of which are vital for confirming reproducibility in organoid research. Regulatory oversight and transparency are essential, but standardized consent forms that safeguard patient rights while enabling collaboration could be a practical solution. Examples from large publicly traded companies (e.g., 23andMe) and biorepositories, like Lifelines Biobank and Cancer Research UK, demonstrate that these challenges can be successfully navigated through organized efforts backed by dedicated funding. The FDA should consider similar large-scale initiatives to streamline these processes and foster reproducibility. Without such measures, the field risks entering not just a reproducibility crisis, but a reality where it becomes nearly impossible to validate findings across studies.
- Incompleteness effect: Organoids often lack the full cellular diversity of living tissues. The addition of immune cells, endothelial cells or stromal cells into organoid culture can more closely mimic living tissue environments. These models, termed co-culture systems, come with their own unique considerations though – namely, the need for isogenic cell types and the assessment of risk inherent in adding another layer of variability. While it is imperative to push towards the most accurate model possible of real-life disease, it is worthwhile considering just how much complexity is actually necessary to obtain an accurate indication of therapeutic response. Computational analyses can guide these decisions, objectively testing model fidelity and ensuring that complexity is added where it genuinely enhances relevance.
- Superficial biomimicry effect: In the quest to replicate the spatial organization of cells, scientists have pushed the boundaries of complexity with modern technologies such as 3D printing. These methods have successfully mimicked tissue structures and even physiological forces such as stretching, shearing and pulling (e.g., gut peristalsis and blood flow). However, these advancements come at a cost: low throughput and limited scalability. Additionally, while visually impressive, the potential fallacy of this approach is that it prioritizes aesthetics over function. True disease modeling should emphasize biological functionality over superficial resemblance.
- Barrier to entry effect: Organoid culture is expensive and requires sophisticated handling. This can increase temptations to cut corners in media quality and experimentation – for example, performing fewer experiments on fewer organoid lines, decreasing sample diversity and rigor. Streamlining minimalistic media recipes and experimental designs can help balance cost with model fidelity.
- Sampling effect: Small sample sizes and limited replicates undermine the reliability of experimental results. Large biobanks with diverse, well-annotated patient samples – representative of the disease heterogeneity observed in Phase 3 clinical trials – are critical for robust experimentation. These biobanks not only enable reproducible studies but also provide a foundation for aligning preclinical models with real-world clinical scenarios. Accurate sampling of diseased PDOs, annotated for clinical behavior, can guide clinical trial design by ensuring that the right drugs are tested on the right patient populations. This approach is also central to advancing precise personalized medicine, aligning therapeutic strategies with individual patient profiles for maximum efficacy.
These are inconvenient truths that everyone in the field must face. There is a clear and broad consensus that without a better approach, we risk entering another era of “reproducibility crisis” potentially worse than the one before. At the University of California (UC) San Diego, we saw this as our slingshot moment: to move forward, we first had to step back, acknowledge limitations and address them with more than just incremental changes. We needed a precise approach to propel us forward. This was the vision behind HUMANOID™.
How HUMANOID is leveraging BioDESIGN to overcome these challenges
HUMANOID is an organized research unit and the home of a handpicked group of young scientists dedicated to advancing organoid research. Its tagline, “Meet us at phase zero,” speaks to its singular mission: advancing preclinical drug discovery with precise human-like models (Figure 1). Housed within the Institute for Network Medicine, HUMANOID is ground zero for an integrated engineering–biology approach called BioDESIGN: biology-driven innovation for drug efficacy, safety and integrated next-gen.
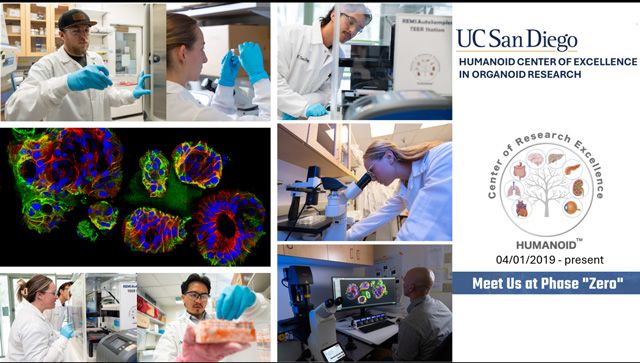
Figure 1: UC San Diego HUMANOID’s scientists at work. Credit: Kyle Dykes.
HUMANOID works collaboratively with other transdisciplinary centers within the Institute for Network Medicine, fostering the kind of cross-pollination that fuels innovation and keeps us focused on our mission: modeling the invariant features of any disease. These invariants are defined as universal, unchanging features that define health or disease in tissues or organs. They represent outcome-deterministic disease drivers, making them ideal targets for therapeutic intervention. A computational arm, the Center for Precision Computational Systems Network (PreCSN), works closely with HUMANOID and enables us to sift through patient tissue data and isolate key disease-driving features that consistently impact outcomes such as complications, therapeutic responses or disease progression. By focusing on invariants, we ensure the outcomes we observe in models are both relevant and reliable in the real world. HUMANOID then focuses on developing and applying organoids and other human primary cells to model disease, predict drug responses and perform real-time toxicity assessments. Within this framework, BioDESIGN addresses the two major issues – lack of efficacy and unmanageable toxicity – responsible for nearly 90% of drug development failures.
Numerous publications showcase how BioDESIGN’s modules rigorously define, recapitulate and measure the fundamental molecular and cellular drivers of health and disease, as well as discover precise targets and biomarkers and guide the assessment of a drug’s therapeutic impact.12,14-25 Because experiments are only as powerful as their controls, BioDESIGN’s principles call for toxicity and efficacy studies to be conducted on both healthy and diseased PDOs. Disease heterogeneity and patient diversity must be captured at the “in a dish” level before relevant therapies can be accurately scaled up to advance to the clinic. By infusing objectivity into the drug development process, BioDESIGN seeks to achieve rigor, standardization and precision, while simplifying models to capture what matters most for any given disease. Its adaptable modular framework can be readily and rapidly applied to diverse therapeutic areas. This was evidenced most recently to address urgent questions in viral immune response during the COVID-19 pandemic.16 This method has since been used reproducibly by others, solidifying its potential to establish drug discovery as a scalable, repeatable process. BioDESIGN is engineered to redefine drug discovery at scale, aiming to set a new standard for adoption by the U.S. FDA in bringing safe, effective therapies to patients faster than ever before.
What makes HUMANOID unique?
- Diversity: We model diverse demographics and age ranges, from womb to tomb, including maternal–fetal systems.
- Therapeutic areas: We prioritize diseases that lack reliable animal models or effective treatments, such as pre-cancer syndromes, fibrotic diseases and inflammation-driven conditions.
- Relevance: Patients are followed prospectively to ensure that the modeled features serve as clinically significant indicators of therapeutic failure, disease progression or complications.
- Accessibility and affordability: We aim to make organoid models affordable and reproducible by using cost-effective media and additives proven to enhance cross-laboratory reproducibility.
- Experts-in-the-loop: We collaborate with top domain experts to ensure that models are relevant to specific therapeutic areas.
By incorporating these principles, HUMANOID has positioned itself as a transformative force in organoid research. The center’s focus on precision, diversity and accessibility has redefined how organoid-based models can be used to address previously unmet needs in drug discovery and translational medicine.
And so, we return to our tagline: “Meet us at phase zero.” It is more than a slogan – it is a thoughtful attempt to inspire and challenge scientists to achieve reproducibility in what is inherently a complex science fraught with imprecision. HUMANOID aspires to solve the problem of overwhelmingly expensive late-stage efficacy failures in drug discovery permanently. At HUMANOID, we believe that this commitment to precision is not just aspirational, but essential for shaping the future of medicine.
1. Sun D, Gao W, Hu H, Zhou S. Why 90% of clinical drug development fails and how to improve it? Acta Pharm Sin B. 2022;12(7):3049-3062. doi:10.1016/j.apsb.2022.02.002
2. McManus R. Ex-director Zerhouni surveys value of NIH research. NIH Rec. 2013;65(13).
3. Molinski SV, Ahmadi S, Ip W, et al. Orkambi® and amplifier co‐therapy improves function from a rare CFTR mutation in gene‐edited cells and patient tissue. EMBO Mol Med. 2017;9(9):1224-1243. doi:10.15252/emmm.201607137
4. Sinha G. This scientist is building miniature guts, livers, and lungs that could save your life one day. Science AAAS, https://www.science.org/content/article/scientist-building-miniature-guts-livers-and-lungs-could-save-your-life-one-day. Published Aug 23, 2017. Accessed Jan 27, 2025.
5. Li X, Francies HE, Secrier M, et al. Organoid cultures recapitulate esophageal adenocarcinoma heterogeneity providing a model for clonality studies and precision therapeutics. Nat Commun. 2018;9(1):2983. doi:10.1038/s41467-018-05190-9
6. Bailey J, Thew M, Balls M. An analysis of the use of animal models in predicting human toxicology and drug safety. 2014;42(3):181-199. doi:10.1177/026119291404200306
7. Shinozawa T, Kimura M, Cai Y, et al. High-fidelity drug-induced liver injury screen using human pluripotent stem cell–derived organoids. Gastroenterology. 2021;160(3):831-846.e10. doi:10.1053/j.gastro.2020.10.002
8. Zhang CJ, Meyer SR, O’Meara MJ, et al. A human liver organoid screening platform for DILI risk prediction. J Hepatol. 2023;78(5):998-1006. doi:10.1016/j.jhep.2023.01.019
9. Paul R. Text - S.5002 - 117th Congress (2021-2022): FDA Modernization Act 2.0. Congress.gov. https://www.congress.gov/bill/117th-congress/senate-bill/5002/text. Published Sept 29, 2022. Accessed January 26, 2025.
10. Matthews JM, Schuster B, Kashaf SS, et al. OrganoID: A versatile deep learning platform for tracking and analysis of single-organoid dynamics. PLoS Comput Biol. 2022;18(11), e1010584. doi:10.1371/journal.pcbi.1010584
11. Soriano-Baguet L, Brenner D. Metabolism and epigenetics at the heart of T cell function. Trends Immunol. 2023;44(3):231-244. doi:10.1016/j.it.2023.01.002
12. Tindle C, Fonseca AG, Taheri S, et al. A living organoid biobank of patients with Crohn’s disease reveals molecular subtypes for personalized therapeutics. Cell Rep Med. 2024;5(10):101748. doi:10.1016/j.xcrm.2024.101748
13. Kim D, Park J, Park HC, et al. Establishment of tumor microenvironment-preserving organoid model from patients with intracranial meningioma. Cancer Cell Int. 2024;24(1):36. doi:10.1186/s12935-024-03225-4
14. Sahoo D, Swanson L, Sayed IM, et al. Artificial intelligence guided discovery of a barrier-protective therapy in inflammatory bowel disease. Nat Commun. 2021;12(1):4246. doi:10.1038/s41467-021-24470-5
15. Katkar GD, Sayed IM, Anandachar MS, et al. Artificial intelligence-rationalized balanced PPARalpha/gamma dual agonism resets dysregulated macrophage processes in inflammatory bowel disease. Commun Biol. 2022;5(1):231. doi:10.1038/s42003-022-03168-4
16. Tindle C, Fuller M, Fonseca A, et al. Adult stem cell-derived complete lung organoid models emulate lung disease in COVID-19. eLife. 2021;10:e66417. doi:10.7554/eLife.66417
17. Li J, Xu X, Tiwari M et al. SPT6 promotes epidermal differentiation and blockade of an intestinal-like phenotype through control of transcriptional elongation. Nat Commun. 2021;12(1):784. doi:10.1038/s41467-021-21067-w
18. Vo DT, Fuller MR, Tindle C et al. SPT6 loss permits the transdifferentiation of keratinocytes into an intestinal fate that resembles Barrett's metaplasia. iScience. 2021;24(10):103121. doi:10.1016/j.isci.2021.103121
19. Sayed IM, Vo DT, Alcantara J et al. Molecular signatures for microbe-associated colorectal cancers. bioRxiv. 2024;2024.05.26.595902. doi:10.1101/2024.05.26.595902
20. Ghosh P, Swanson L, Sayed IM et al. The stress polarity signaling (SPS) pathway serves as a marker and a target in the leaky gut barrier: implications in aging and cancer. Life Sci Alliance. 2020;3(3):e201900481. doi:10.26508/lsa.201900481
21. Sinha S, Alcantara J, Perry K et al. Machine-learning identifies a strategy for differentiation therapy in solid tumors. bioRxiv. 2024;2023.09.13.557628. doi:10.1101/2023.09.13.557628
22. Sinha S, Castillo V, Espinoza CR et al. COVID-19 lung disease shares driver AT2 cytopathic features with Idiopathic pulmonary fibrosis. EBioMedicine. 2022;82:104185. doi:10.1016/j.ebiom.2022.104185
23. Sayed IM, Tindle C, Fonseca AG, Ghosh P, Das S. Functional assays with human patient-derived enteroid monolayers to assess the human gut barrier. STAR Protoc. 2021;2(3):100680. doi:10.1016/j.xpro.2021.100680
24. Sayed IM, El-Hafeez AAA, Maity PP, Das S, Ghosh P. Modeling colorectal cancers using multidimensional organoids. Adv Cancer Res. 2021;151:345-383. doi:10.1016/bs.acr.2021.02.005
25. Sinha S, McLaren E, Mullick M, Singh S, Boland BS, Ghosh P. FORWARD: A learning framework for logical network perturbations to prioritize targets for drug development. bioRxiv. 2024:2024.07.16.602603. doi:10.1101/2024.07.16.602603